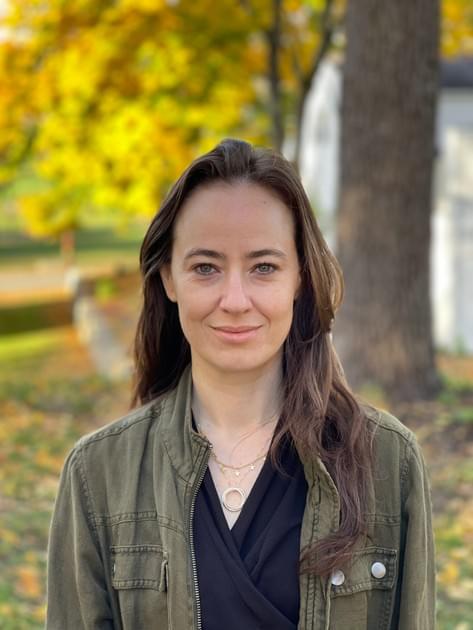
> 400
Logged open water SCUBA dives.
8
Digits of π (pi) I have memorized.
2
My Erdös number. I don't have a Bacon number... yet.
Here are a few of my talks & panels:
Something Digital is an annual festival curated by Bright Humans in collaboration with some of Australia's, and the world's, greatest digital minds. For the 2021 edition, the organizers asked me to share a provocative thought with their audience, so I climbed on my proverbial soap box: Data Is Not Ground Truth
In 2021, I shared with the audience at SheData a few pieces of insight gathered over many years of data work. I talked about my experience in building Data Science teams and the challenges they face, as well as other lessons learned throughout my career.
On this 2020 episode of Gradient Dissent, with Lukas Biewald, I'm joined by my colleague Danielle Dean to share a bit about how we make machine learning work at scale at iRobot.
I presented my point-of-view on managing teams of data scientists at the RStudio::Conf 2019 in Austin, TX. More info and slides here.
Also while at RStudio::Conf 2019 in Austin, TX, I was glad to join a stellar panel of data science veterans—Hilary Parker of StitchFix, Karthik Ram from UC Berkeley, and TracyTeal of Data Carpentries, moderated by Eduardo Ariño de la Rubia of Instagram—to discuss Growth and Data Science: Individuals, leaders, organizations and responsibilities. We talked about growing as data scientists, as data science leaders, and as data science teams.
In early 2018 Google hosted MassTLC's Applied Machine Learning Developer Day, where I presented a talk ‘On Keeping Things Simple’, about deploying machine learning algos in production.
You can see the slides and a few additional resources here.
This is the same presentation I gave as a keynote at GE's Industrial Machine Learning Workshop in late 2017 during the Minds + Machines conference.
In this talk from 2017's edition of ODSC East, I discussed strategies for building and maintaining successful data science teams in a corporate setting, sharing common mistakes and errors that folks and companies can make when setting out to build data science teams.
I push back against myths such as “Unicorns” and “Super-chickens” that hurt companies and their data science teams, and provide those working in the industry frameworks to achieve a better ROI from data science, especially those in charge of hiring data professionals.
On what must have been the hottest NYC summer day of 2017, Columbia University’s Tow Center for Digital Journalism and Brown Institute for Media Innovation presented Artificial Intelligence: Practice and Implications for Journalism. The conference featured leading journalists, technologists, legal scholars and academics in conversation around the current and near future applications, challenges, and legal implications of AI implementation in products and newsrooms.
In May 2017 I gave a talk on building data science teams in industry and setting them up for success at csv,conf—a non-profit community conference run by some folks who really love data and sharing knowledge.
You can see the slides and a few additional resources here.
I also gave this presentation at ODSC East 2017, where it ranked among the top 3 rated presentations; you can watch it here.
The WithTheBest team organizes several tech conferences every year, and I was happy to discuss my experience with deploying Machine Learning in Production alongside such great a speaker line-up in their September 2016 AI conference.
Each season Design Museum Boston organizes a UNITE event, an amazing fusion of themes and ideas. The Spring 2015 event brought various Boston-based communities together to focus on Data Visualization.
The 2014 Boston Data Conference, held lots of great and relevant sessions. In mine I discuss how to build a quantitative story and tell it with data.
Here are a few appearances in audio & print:
Webinar, September 2022
Anaconda conducted its State of Data Science survey to gather demographic information about our community, ascertain how that community works, and collect insights into big questions and trends that are top of mind within the community. The resulting report looks at actionable issues within the data science, machine learning, and artificial intelligence industries, like open-source security, the talent dilemma, ethics and bias, and more.
I joined Peter Wang, co-founder, and CEO of Anaconda to explore the survey’s findings, what the future holds, and the general State of Data Science in 2022.
Analytics Power Hour, June 2022
Every consumer is now aware, at some level, that they are constantly generating data simply by moving through the world. And, every organization that puts physical devices or digital experiences into the paths of consumers has to make decisions about what data they collect, how they manage it, and what they do with it (both the immediate plans and what unknown plans may emerge in the distant future). The questions, decisions, and mindsets that this reality brings into play are just one big gray area after another. I grappled with these issues on a daily basis both professionally and personally, and thoroughly enjoyed this lively and thought-provoking discussion on the subject with Mike, Moe, and Tim.
Experiencing Data, December 2019
In this episode of the Experiencing Data podcast, I join host Brian O'Neill to discuss math-washing, becoming a data strategist, and the role data science plays in robotics. We also chat about the division between technical and non-technical skills, what data breadcrumbs are and what you should know about them, the skills I believe matter most when turning data science into a producer of decision support, why the last mile of the UX is often way longer than one mile, and the critical role expectation management plays in data science.
The Experiencing Data podcast reveals the strategies and activities that product, data science and analytics leaders are using to deliver valuable user experiences that drive business value. In each episode, Brian explores his guests' expertise, experiences, and lessons learned while trying to turn advanced analytics, ML, and AI capabilities into simple, engaging, useful software applications for people.
This Week in Machine Learning & AI (TWIMLAI), August 2019
It was a treat to tell Sam all about Data Science at iRobot. When we recorded the episode, iRobot was just completing an internal re-aligning; in our conversation we covered our freshly minted architecture and I shared some insight into a new data-forward thought process—and a look at the evolution of iRobot on that front. We also discussed where iRobot data comes from, how we taxonomize data science, the platforms and processes that have been put into place to support delivering models in production, and the role of DevOps in bringing these various platforms together.
TWiML & AI, hosted by Sam Charrington, is a podcast that brings top minds and ideas from the world of AI to a broad and influential community of data scientists, engineers and tech-savvy business and IT leaders.
Masters of Data, June 2019
iRobot is one of the most innovative robotics companies on the planet, and in this episode I talked about how iRobot's recent product announcements reveal more than just product innovation. I discussed our new iRobot 2.0 platform and our new approach to building robots, developing software, and sciencing on data.
Masters of Data is a podcast sponsored by Sumo Logic in which host Ben Newton talks to the people that are driving the Modern Data revolution and hear their stories about Data Science, Ethics, Privacy, Innovation, Cool Technology, and more.
ZDNet, May 2019
This article, by Larry Dignan for Between the Lines, details how iRobot has used its new design, software, and data science strategies to expand into new areas, using an approach to the smart home that is different from its big tech rivals. "Cultural change is why we are spending money on data science," CEO Colin Angle says. "iRobot is transitioning from a largely hardware company to one about data."
ZDNet and TechRepublic are online magazines that bring together global news coverage and analysis on local and global IT industry trends and opportunities, and is a part of CBS Interactive.
DataFramed, November 2018
In this episode, Hugo and I discuss managing data science teams: we talk about how to get into data science management, how to hire data scientists, and much more.
DataFramed is hosted by Hugo Bowne-Anderson, who explores what problems data science tries to solve instead of what definition fits it best—from automated medical diagnosis and self-driving cars to recommendation systems and climate change.
DataFramed is a podcast from DataCamp. DataCamp's executive team has acknowledged sexual assault and has tried to limit the reach of this acknowledgement. The company also quickly terminated employees who raised ethical concerns about the cover-up even as it took over 500 days to address it publicly. I did not know the extent of this when I recorded this episode, so I'm now using this space to help search engines find and index these links.
At first I thought it was a giant missed opportunity by DataCamp to do the right thing from the start, but it is hard to continue to assume positive intent after all of these actions have come to light. Please do not give money to DataCamp, you can find many alternatives in this excellent thread by Chris Engelhardt.
Harvard Business Review, October 2018
Many managers of data science teams become managers because they were great individual contributors and not necessarily because they have the skills or training to lead a team. But management is a skill unto itself, and relying on your experience as a successful individual contributor is not enough to ensure that you are able to retain and develop great talent while delivering valuable learnings, products, and outcomes back to the organization.
Great data scientists have career options and won’t abide bad managers for very long. If you want to retain great data scientists you’d better commit to being a great manager. In this article I discuss what does it take to become a great manager: caring about your team members, connecting their work to the business, and designing diverse, resilient, high-performing teams.
GE Industrial Machine Learning Workshop, October 2017
I gave a keynote presentation at the first GE Industrial Machine Learning Workshop on October 24, 2017 in San Francisco, CA during GE's Minds + Machines 2017 event. In it I discussed how, in the field of data science, we are constantly bombarded with innovative approaches and methods. We can even fall prey to our impulses of following the latest and coolest at the expense of our objectives. When does it make sense to deploy complex solutions into production environments? And how should we assess the pros and cons of doing so?
You can see the slides and a few additional resources here. The keynote wasn't recorded, but I gave a very similar talk at the MassTLC Applied Machine Learning Developer Day 2018 (see recording above).
The Rest of Us in Tech Podcast, July 2017
In this episode, Angela and Sarah talk about data science and robots, how awesome Wonder Woman is, and crack up laughing at silly super powers.
The Rest of Us in Tech by Sarah Withee is a podcast that includes different people in tech: women, people of color, LGBTQ people, disabled, and more who are developers, designers, managers, artists, writers, or anyone who feels they exist in a technology space.
Hanselminutes Podcast, June 2017
In this episode Angela sits down with Scott and demystifies the major concepts. Is this a new scienceand an old one? What's the traditional path for a Data Scientist - and is that the only path?
The Hanselminutes Podcast by Scott Hanselman is like Fresh Air for Developers: a deep tech talk from an inclusive perspective.
Links from the show:
Women in Data Science Cambridge, February 2017
The Global Women in Data Science (WiDS) Conference aims to inspire and educate data scientists worldwide, regardless of gender, and support women in the field. This annual one-day technical conference provides an opportunity to hear about the latest data science related research and applications in a broad set of domains. For the 2017 conference, over 80 events were held across the globe, and I got to share my experiences and insights from deploying various systems, presenting perspectives from both academia and industry at the WiDS Cambridge event hosted by Harvard University SEAS, MIT, and Microsoft Research, New England.
Thinking Poker Podcast, February 2017
I am an occasional poker player with an interest in Libratus and its implications for artificial intelligence. In this interview (starting 41 minutes into the recording), we talked about poker’s intersections with data science and artificial intelligence, as well as what’s going through a data scientist’s mind when they sit down for beer and poker with friends.
The Thinking Poker Podcast is a weekly conversation between Andrew Brokos and Nate Meyvis about strategy, review books, and explore the culture of poker.
Wall Street Journal, December 2016
Artificial intelligence is having a moment. Startups that claim to be using AI are attracting record levels of investment. Big tech companies are going all-in, draining universities of entire departments. But wringing measurable utility from these new AI toys can be hard. I chat with Chris Mims about my thoughts on what's next for AI in the near- and mid-term.
Dataconomy and WTB Medium, July 2016
Since 2014, AI startups have experienced an explosion of funding, giving even more incentive to the keen computer scientist. In essence, programming computers to think for themselves may just be the ultimate goal. I did a Q&A in which I discussed the challenges is making sense out of the unbelievable complexity of our data: weather models, consumption tracking, tariff structures, billing details, geographic nuances, local and global regulations, sector and industry benchmarks, macro trends, and much more. I review how the data science team turns all of that “messy data” into information that is useful, valuable, and actionable for our customers.
Slice of MIT, November 2015
Over 90 percent of all of the data in the world was created in the last two years and it's our job to figure out what that might mean. In this interview I talk about my path to data science, and how “I’ve always used data to try and explain what is happening, why it’s happening, and when it will happen again.”
Payette, March 2015
Whether we are trying to convey building program or energy performance, we utilize graphic representations of data to tell complex stories. Data visualization is a universal tool. The key is to remember the intent and decide the narrative before you create the end product because not all data is actually telling you what you think, you have to dig a little deeper.